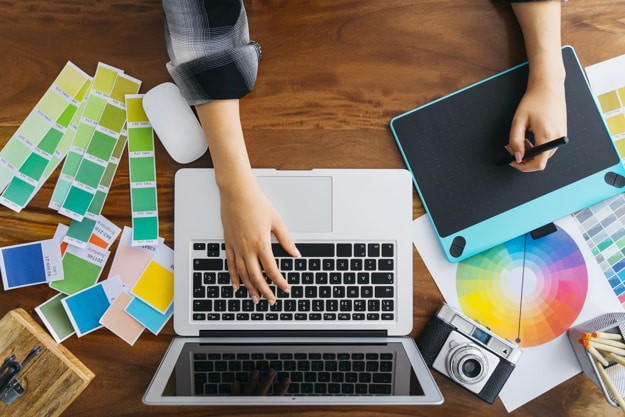
A/B testing, also known as split testing, is a popular method of comparing two versions of a web page, an email, or any other marketing asset to see which one performs better. A/B testing can help marketers and designers make data-driven decisions and improve the user experience (UX) and conversion rates of their products.
However, A/B testing can also be challenging and time-consuming. It requires a lot of manual work, such as creating hypotheses, designing variants, setting up experiments, analyzing results, and implementing changes. It also requires a sufficient amount of traffic and conversions to reach statistical significance and avoid false positives or negatives.
This is where artificial intelligence (AI) can help. AI is a branch of computer science that aims to create machines or systems that can perform tasks that normally require human intelligence, such as learning, reasoning, and problem-solving. AI can enhance the A/B testing process by automating some of the tasks, generating new ideas, and optimizing the results.
In this article, we will explore how AI can be used in A/B testing to optimize design and UX for conversions. We will cover the following topics:
- How AI can automate and improve the A/B testing process
- How AI can generate new and original variants based on user input or data analysis
- How AI can enhance the creativity and productivity of human designers by providing them with suggestions, feedback, and inspiration
- Some examples and case studies of successful AI-powered A/B testing
How AI can automate and improve the A/B testing process

One of the main benefits of using AI in A/B testing is that it can automate some of the tedious and repetitive tasks involved in the process, such as:
- Image resizing, color correction, and layout generation
- Experiment setup, execution, and monitoring
- Data collection, analysis, and reporting
By automating these tasks, AI can save time and resources for marketers and designers, allowing them to focus on more strategic and creative aspects of their work. AI can also improve the quality and accuracy of the A/B testing process by using advanced algorithms and techniques, such as:
- Machine learning: Machine learning is a subset of AI that enables machines to learn from data and improve their performance over time. Machine learning can help A/B testing by finding patterns and insights from large amounts of data, such as user behavior, preferences, feedback, and conversions. Machine learning can also help A/B testing by adapting to changing conditions and environments, such as user segments, devices, platforms, or seasons.
- Evolutionary algorithms: Evolutionary algorithms are a type of machine learning that mimic the process of natural selection and evolution. Evolutionary algorithms can help A/B testing by creating and testing multiple variants simultaneously, rather than sequentially. Evolutionary algorithms can also help A/B testing by selecting the best-performing variants based on predefined criteria or objectives, such as conversion rate or revenue. Evolutionary algorithms can also help A/B testing by combining or mutating the best-performing variants to create new ones that may perform even better.
- Predictive analytics: Predictive analytics is a type of machine learning that uses historical data to make predictions about future outcomes or events. Predictive analytics can help A/B testing by estimating the potential impact or value of each variant before launching an experiment. Predictive analytics can also help A/B testing by forecasting the optimal duration or sample size for each experiment to reach statistical significance.
See Too: https://gjsmart2023.fun/?p=116
How AI can generate new and original variants based on user input or data analysis
Another benefit of using AI in A/B testing is that it can generate new and original variants based on user input or data analysis. This can help marketers and designers overcome the limitations of their own intuition or creativity and discover new possibilities for improving design and UX.
Some examples of how AI can generate new variants are:
- Natural language processing (NLP): NLP is a subset of AI that enables machines to understand and generate natural language, such as text or speech. NLP can help A/B testing by creating new variants based on text descriptions provided by users or marketers. For example, an NLP system could create a new headline for a landing page based on a text prompt such as “How to increase your sales in 30 days”.
- Computer vision (CV): CV is a subset of AI that enables machines to understand and generate visual content, such as images or videos. CV can help A/B testing by creating new variants based on images provided by users or marketers. For example, a CV system could create a new logo for a brand based on an image prompt such as “A blue bird with wings spread”.
- Generative adversarial networks (GANs): GANs are a type of machine learning that use two competing neural networks to generate realistic content. GANs can help A/B testing by creating new variants based on data analysis or existing content. For example, a GAN system could create a new product image based on the analysis of user preferences or feedback.
How AI can enhance the creativity and productivity of human designers by providing them with suggestions, feedback, and inspiration

A third benefit of using AI in A/B testing is that it can enhance the creativity and productivity of human designers by providing them with suggestions, feedback, and inspiration. AI can act as a partner or a coach for human designers, rather than a replacement or a competitor.
Some examples of how AI can enhance human creativity and productivity are:
- Suggestions: AI can provide human designers with suggestions for improving their variants based on data analysis or best practices. For example, an AI system could suggest changing the color, size, or position of a call-to-action button based on the analysis of user behavior or conversions.
- Feedback: AI can provide human designers with feedback on their variants based on data analysis or user feedback. For example, an AI system could provide a score or a rating for each variant based on the criteria or objectives defined by the user or the marketer.
- Inspiration: AI can provide human designers with inspiration for creating new variants based on data analysis or existing content. For example, an AI system could provide a list of keywords, images, or headlines that are relevant or popular for the target audience or the topic.
Some examples and case studies of successful AI-powered A/B testing
To illustrate how AI can be used in A/B testing to optimize design and UX for conversions, here are some examples and case studies of successful AI-powered A/B testing:
- Bannersnack: Bannersnack is an online platform that allows users to create professional-looking banners and ads. Bannersnack used Hotjar’s heatmaps to analyze how users interacted with their landing page and identify areas for improvement. Bannersnack then used evolutionary algorithms to create and test multiple variants of their landing page simultaneously, rather than sequentially. Bannersnack was able to increase their sign-ups by 25% by using AI-powered A/B testing.
- Adobe: Adobe is a software company that offers various creative products and services. Adobe used predictive analytics to estimate the potential impact of each variant before launching an experiment. Adobe also used machine learning to optimize the duration and sample size of each experiment to reach statistical significance faster. Adobe was able to increase their conversions by 20% by using AI-powered A/B testing.
- DALL·E: DALL·E is an AI system developed by OpenAI that can create images from text descriptions. DALL·E used natural language processing and computer vision to generate new and original images based on text prompts provided by users or marketers. DALL·E was able to create images that are realistic, abstract, or surreal based on the user’s input.
Summary
AI in A/B testing is a powerful way to optimize design and UX for conversions. AI can help automate and improve the A/B testing process, generate new and original variants based on user input or data analysis, and enhance the creativity and productivity of human designers by providing them with suggestions, feedback, and inspiration.
Some of the benefits of using AI in A/B testing are:
- Saving time and resources
- Improving quality and accuracy
- Overcoming limitations of intuition or creativity
- Discovering new possibilities for improvement
- Increasing conversion rates and revenue
Some of the challenges of using AI in A/B testing are:
- Choosing the right tools and platforms
- Defining clear criteria and objectives
- Interpreting and implementing the results
- Balancing human and machine input
- Ensuring ethical and responsible use
Some of the examples and case studies of successful AI-powered A/B testing are:
- Bannersnack: increased sign-ups by 25% by using evolutionary algorithms
- Adobe: increased conversions by 20% by using predictive analytics and machine learning
- DALL·E: created realistic, abstract, or surreal images from text descriptions by using natural language processing and computer vision